How Finarb's Predictive Model helped a leading patient financial solutions provider ensure efficient claims management
Project Objective
As a revenue cycle management vendor, the client wished to deploy predictive modeling to assess the likelihood of claims getting invoiced, understanding drivers of claim denials, and deploy mitigation strategies, thereby ensuring efficient claims management for seamless patient experience and maximized revenue recovery for healthcare providers.
Approach
To train the machine learning model, we used data about patient admissions, census data, and mortality rates to model the likelihood of claims getting invoiced. The dataset was further enhanced by incorporating mortality rates of various diseases, providing additional context that could influence the invoicing of claims. Moreover, engineered features such as the season, day of the week, and time since the last visit were added to capture historical patterns in the dataset.
We created two separate models for the inpatient and outpatient cases, which allow us to better understand the different characteristics and patterns. For instance, inpatient cases often involve more complex procedures and longer stays than outpatient cases. In addition, the differences in the care processes can lead to significant differences in costs. These cost differences can influence the likelihood of a claim being invoiced. Having two different models can account for this contrast, and we can draw better conclusions. The relative advantage of our approach is visible when comparing the importance of various features for the two models. On one hand, in the inpatient model, age of the patient, average delay between two visits, and whether the patients commute using public transport are the most important features in determining whether a claim will get invoiced. While in the outpatient model, the average delay between visits, ratio of claims approved, and whether a household makes over $200K annually are the most important features in deciding claims. It would not have been possible to understand these nuances with a single model for both the cases.
Outcome
The inpatient model achieved a training accuracy of 82.16%. The model’s AUC-ROC score of 92.8% indicates its strong ability to distinguish between claims that are likely to be invoiced and those that are not. Similarly, the outpatient model demonstrated a training accuracy of 81.64%. Its AUC-ROC score of 77.3% suggests that though slightly lower than the inpatient model, it still effectively differentiates between claims that will and will not be invoiced. These results underscore the models’ robustness and their potential to enhance revenue cycle management by accurately predicting claim invoicing.
Inpatient Model | Outpatient Model | |
---|---|---|
Training Accuracy | 0.82 | 0.82 |
Training AUC-ROC | 0.93 | 0.77 |
Validation AUC-ROC | 0.83 | 0.75 |
Precision | 0.81 | 0.86 |
Recall | 0.76 | 0.53 |
F1-Score | 0.78 | 0.65 |
Way Forward
Further to this, we will also be developing a patient-responsiveness-to-outreach model to identify the best channels to engage with patients for the high risk claims to ensure fast resolution of their claim denials and increase conversion rates. Additionally Finarb will also develop a robust MLOps pipeline that facilitates seamless model training, hyperparameter tuning and deployment.
Our Client Stories
Leading Public Hospital, Texas
AI in Clinical Decision Support for Early Sepsis Detection

Finarb helped in clinical decision support with an AI-Driven Real-Time Sepsis Monitoring Solution to accurately risk stratify patients who have a high likelihood of developing sepsis within a certain time window based on biomarker levels and vitals, sourcing data from Patient Monitoring Devices and Lab Information Systems.
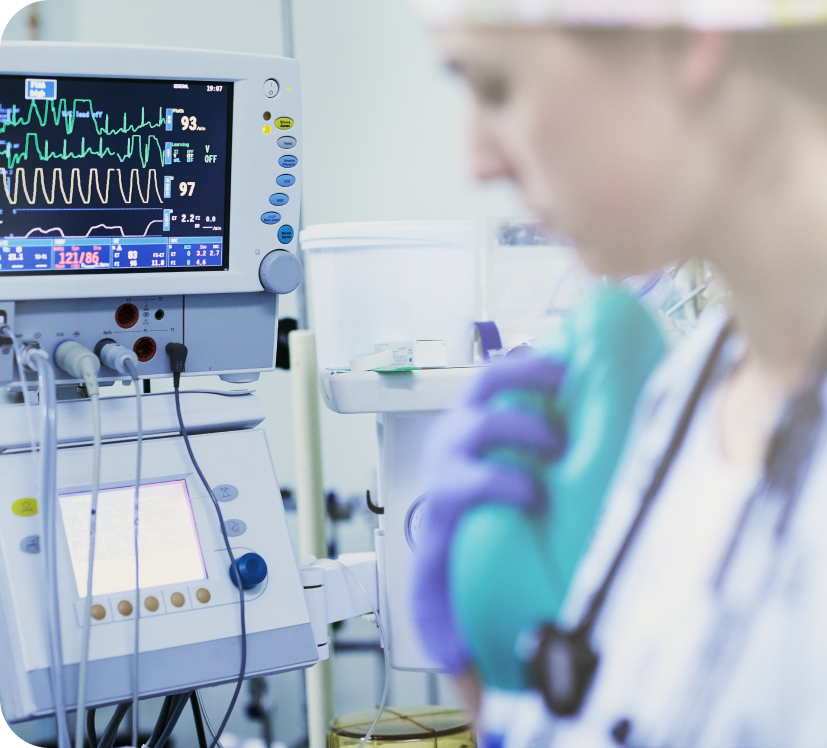
A leading pharmacy support services provider
Using Real World Data in AI driven Medication Adherence

Finarb collaborated with a leading pharmacy support service provider, to develop an AI enabled medication adherence monitoring model, integrating real world data & refill data, to accurately stratify high-risk patients, identify root causes of weak adherence and suggest precision targeted interventions.
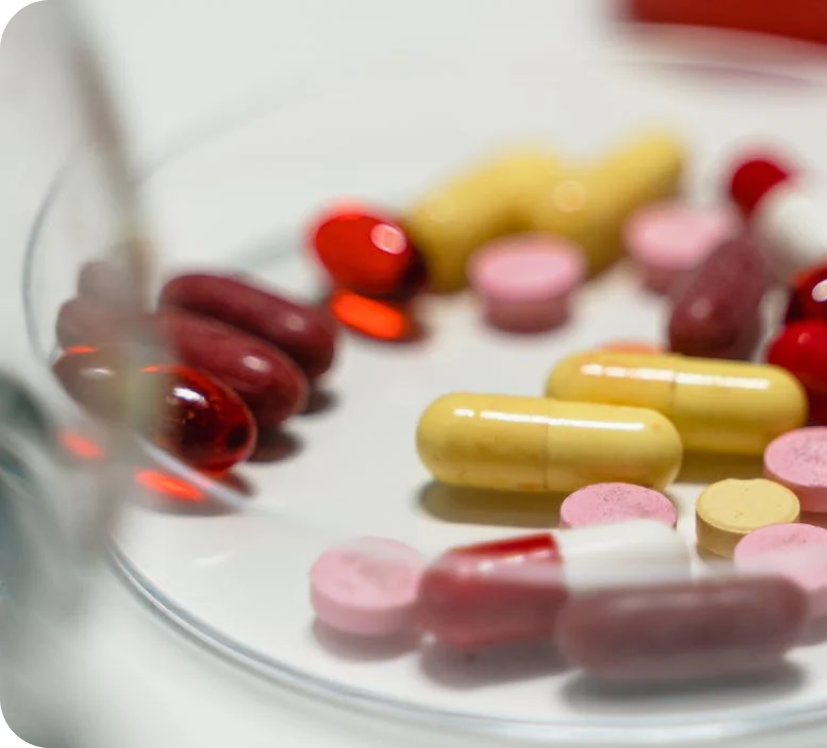
Radiology Clinic Chain, Texas
Tackling patient compliance with secure data warehousing

Finarb built a clinical data warehouse for a leading radiology clinic based in Texas, operating 100+ centers in 13 markets. With data spread across disparate sources, the clinic was struggling with patient compliance and low room utilization. We enabled secure integration of data from multiple structured and unstructured data sources and healthcare IT systems, followed by predictive modelling to identify patients with a high risk of non-compliance and failure points with targeted interventions to improve future compliance.
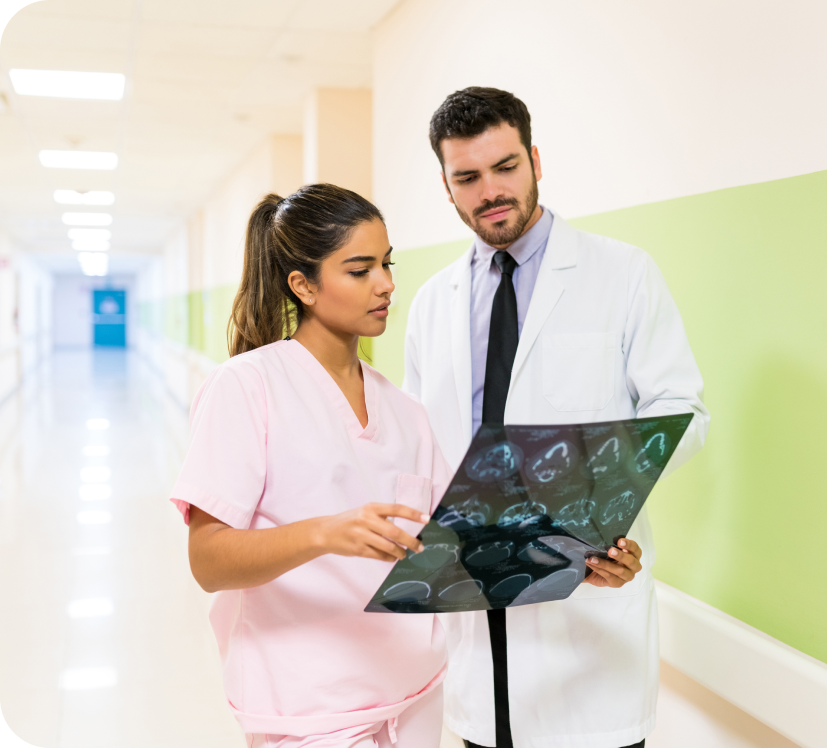