How Finarb's secure data warehousing and AI-enabled patient compliance model helped a leading provider of mammography and imaging services enhance data-driven decision-making
Client’s Business Goals:
- Consolidation of disparate data sources that would provide a comprehensive 360° view of patient data and offer more effective data interpretation.
- Feature engineering and predictive modelling for identifying the top drivers of non-compliance and suggesting customized interventions per patient.
Business Outcome:
- Achieving a comprehensive, integrated perspective of patient data, facilitating data extraction, analysis, and reporting processes.
- Enhanced Patient Monitoring and Data-Driven Insights
- Patient Risk Stratification and identifying high-risk patients for targeted custom interventions to increase compliance
Services Offered
Secure Data Warehousing for building a single point of data truth-
- Data Modelling
- Data Encryption/Decryption
- PHI Protection
- Automated ETL
- Logging and Maintenance.
Predictive Modelling to identify top drivers of non-compliance and suggesting interventions-
- Feature Engineering
- Patient Risk Stratification
- Risk Score Prediction
- Custom Interventions for high-risk patients
Business Challenge that prompted the use of AI
As part of their objective towards early-stage breast cancer detection, the client wanted to measure and improve compliance rates among patients for mammography screenings. Also from an operational aspect, high compliance would mean optimal utilization of rooms and technologists for them. However, with a high volume of patient data residing across disparate sources, it was a time-consuming process to analyse and identify high-risk patients for non-compliance. Without a centralized data repository, processing and analysing data efficiently was a challenge, leading to delays in gaining insights, ineffective patient outreach campaigns and poor patient compliance. Additionally, the lack of integration between different data sources and systems hindered interoperability and data exchange.
The client engaged Finarb Analytics Consulting to address the need for establishing a single point of data truth, measuring patient compliance, and advising interventions to improve the same.
As per a scientific literature published in the National Library of Medicine, women who participated in regular mammography screenings had a statistically significant 41% reduction in breast cancer-related mortality over a 10-year period.
Building a secure data warehouse as a single point of data truth
We conducted several sessions with the client to understand their unique needs and desired insights from their existing data. In addition, we anticipated future data requirements to ensure the planned infrastructure was flexible, scalable and capable of meeting evolving business intelligence requirements and decision support. By building a data warehouse, the data from various sources would be fetched in real time and before storing in the data warehouse, the original data would be reorganised, reprocessed, and appended with different data from other diverse sources.
The client had 3 different sources of data with different schemas for data storage. The first stage in the integration process was data modelling where Finarb identified the data that needed to be captured from several decentralized and diverse sources which contained information related to mammograms, patient portals, radiologist reports, clinical notes, etc. and built a multi-dimensional data model. This database was built using HIPAA compliant Azure Services.
How we implemented ETL and secure data warehousing
The data was extracted and ingested from disparate source databases of the client to Azure maintaining stringent data security measures. Data quality checks were performed to ensure accuracy, consistency, and integrity of the loaded data. This involved data validation checks and unit tests.
The data for building the data warehouse was arranged into several zones depending on the type of data, usage, and access requirements. Each zone denoted a specific and unique location within the data warehouse and had different functionalities and access. These zones provided a robust and structural framework in how the data was collected, stored and used for further business requirements within a data warehouse.
Development of Predictive Model
We integrated the master data with the publicly available NPI registry & census data to bring in additional attributes of the referring physician and the socio-economic indicators of the patient respectively. Additional features were also engineered that enhanced the model's predictive capacity regarding a patient's adherence to annual mammography screening protocols.
Multiple predictive models were trained using the feature engineered dataset to make accurate predictions of patients' likelihood of compliance. This enabled the client to identify high-risk patients who were more likely to be non-compliant and the major drivers of non-compliance for those patients. K-fold cross validation method was used for training and testing the data to improve the accuracy of the model.
We explored a range of advanced algorithms including XGBoost, LightGBM, and Random Forest to construct the model for predicting non-compliance. The results varied depending on the model and the selected input parameters. The model, XGBoost performed well on the dataset and achieved an AUC-ROC of 76%.
Identification of drivers of non-compliance
Based on the findings of the predictive model, Finarb assisted the client in stratifying patients into high, medium, and low-risk segments. This segmentation facilitated targeted interventions and monitoring, optimizing patient compliance rates. We further clustered high-risk patients into an optimal number of sub-segments based on their top drivers of non-compliance. For each such sub-segment the algorithm found common denominator driver(s), which could be used by them to deploy customised interventions specifically tailored for a high-risk sub-segment.
Results and Benefits:
The collaboration between the client and Finarb yielded significant results and benefits:
- Enhanced Patient Monitoring and Data Driven Insights: A secure data warehouse allowed to efficiently track and monitor patient compliance with routine mammogram checks.
- High-resolution dashboards and robust reporting capabilities provided a comprehensive overview of its operations and performance.
- Targeted and Personalized Interventions: The client was able to perform targeted and personalized outreach programs for high-risk patients, optimizing the allocation of resources and improving overall efficiency.
Our Client Stories
Leading Public Hospital, Texas
AI in Clinical Decision Support for Early Sepsis Detection

Finarb helped in clinical decision support with an AI-Driven Real-Time Sepsis Monitoring Solution to accurately risk stratify patients who have a high likelihood of developing sepsis within a certain time window based on biomarker levels and vitals, sourcing data from Patient Monitoring Devices and Lab Information Systems.
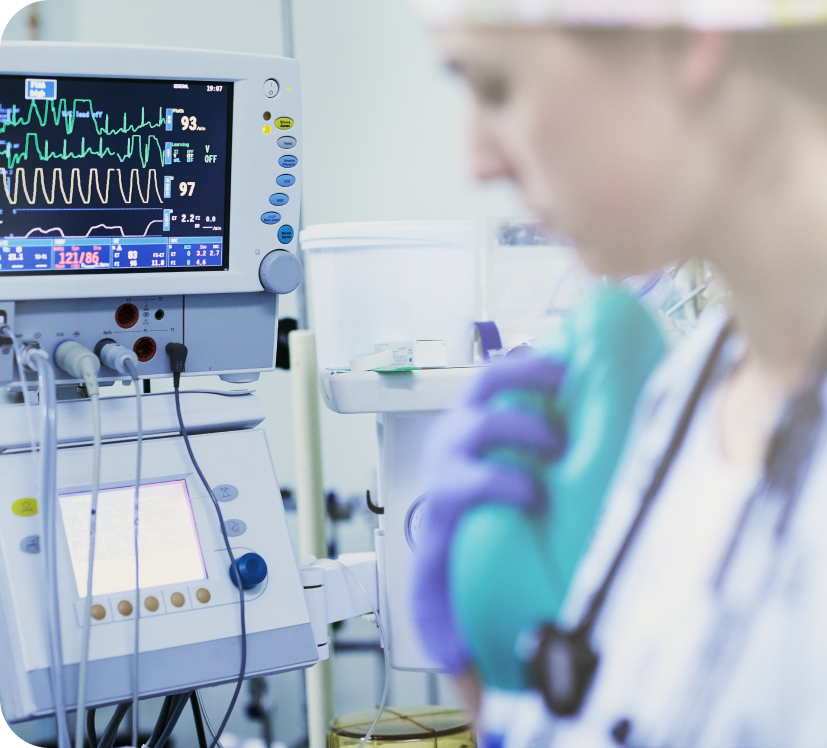
A leading pharmacy support services provider
Using Real World Data in AI driven Medication Adherence

Finarb collaborated with a leading pharmacy support service provider, to develop an AI enabled medication adherence monitoring model, integrating real world data & refill data, to accurately stratify high-risk patients, identify root causes of weak adherence and suggest precision targeted interventions.
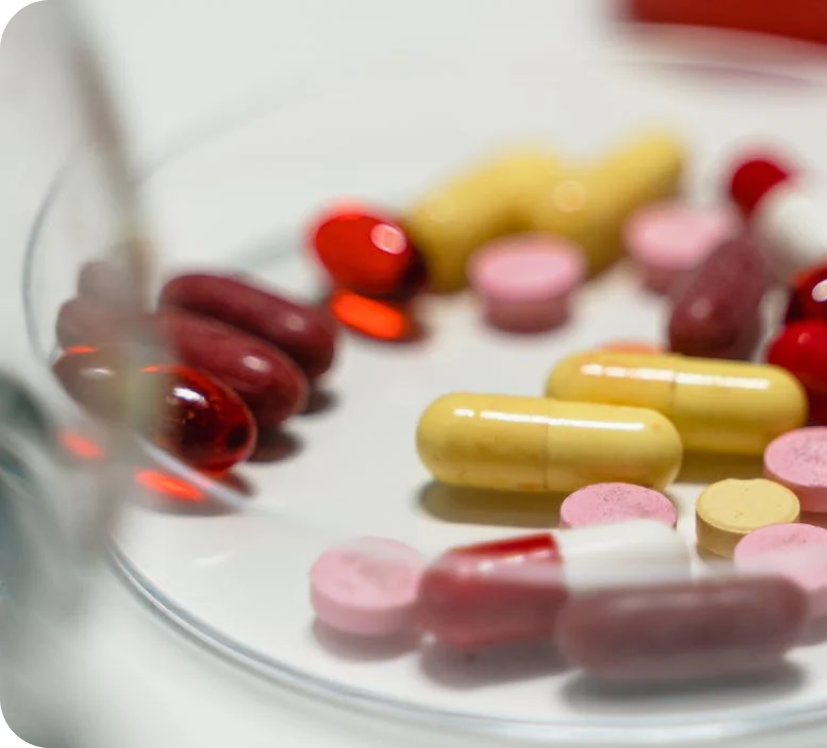
Radiology Clinic Chain, Texas
Tackling patient compliance with secure data warehousing

Finarb built a clinical data warehouse for a leading radiology clinic based in Texas, operating 100+ centers in 13 markets. With data spread across disparate sources, the clinic was struggling with patient compliance and low room utilization. We enabled secure integration of data from multiple structured and unstructured data sources and healthcare IT systems, followed by predictive modelling to identify patients with a high risk of non-compliance and failure points with targeted interventions to improve future compliance.
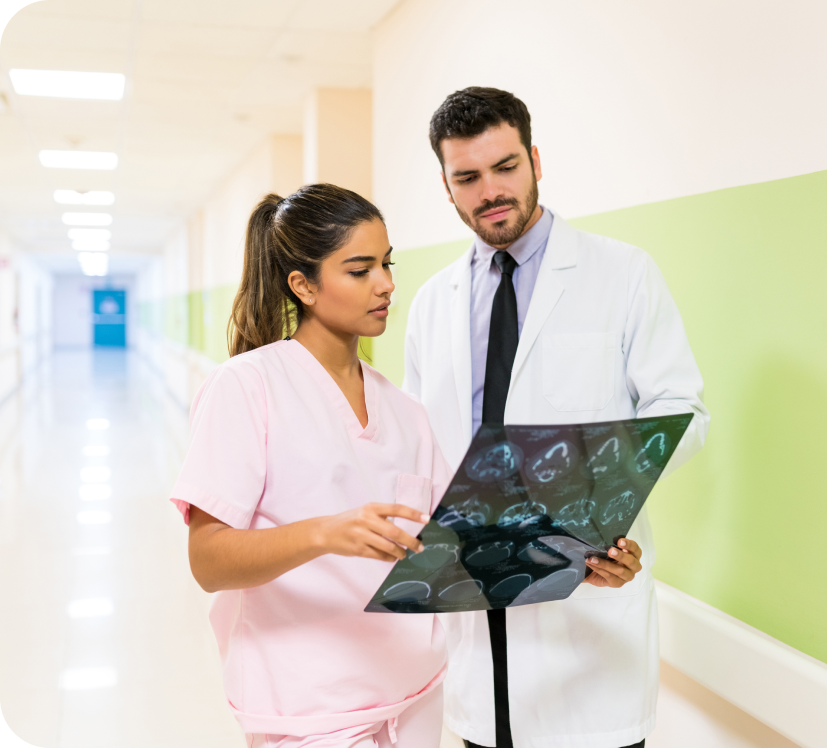