AI Driven Medication Adherence monitoring system enabling improvement in overall medication adherence rates among patients.
Overview
The client being a data driven company, works with its customers and partners, predominantly pharmacies, on providing automated patient care solutions that improves patient experiences and health outcomes. In view of this, they wanted to develop a solution to tackle the challenge of medication non-adherence among patients. Traditional methods deployed for improving adherence produced inconsistent results due to the failure to identify, time, and structure strategies targeted at high-risk patients. Also scaling interventions across large patient-sets is a challenge in traditional methods. This emphasized the need for an AI enabled adherence monitoring model to accurately stratify high risk patients, identify root causes of weak adherence and suggest precision targeted interventions.
The task at hand was to measure and reduce the risk of medication non-adherence on a set of rheumatoid arthritis patients. We determined medication adherence in terms of Proportion of Days Covered (PDC). A patient PDC score ≥0.9 is conventionally considered adherent, and below is considered non-adherent. The task was to build an Adherence Risk Model that provides a probability score of a patient to have a future PDC less than 0.9, thereby stratifying high risk patients, identifying failure points, and mitigating areas of concern.
Clinical Data Inputs and Modelling
We combined real world patient assessment data sourced through primary data collection surveys/questionnaires with medication refill data sourced from pharmacy dispensing databases. North of 1.8 Million patient records were analysed.
We used an exhaustive list of features including patient demographics, prior adherence performance, SDOH data, medication features including drug name, strength, route of administration, etc. For more accuracy and enhanced interpretability, patient sentiment analysis was used to create patients' state-of-being variables. We used NLP techniques for analysing unstructured patient feedback data from follow-up assessments.
We proceeded to assess the importance of these features in predicting medication non-adherence using a ML classifier. The model was trained using the dataset with the engineered features and learned the relationships between the features and medication adherence status and provided a probability score of a patient to have a future PDC less than 0.9 in the upcoming refill.
Further we found the impact and magnitude of each variable on future PDC, specifically if each variable would potentially increase or decrease future PDC, also the magnitude of impact on change in PDC
Risk Stratification of Patients, identifying risk factors for poor adherence & mitigation
Patients were evaluated and categorized into groups based on their predicted adherence levels. The effectiveness of our risk stratification was notably high due to its strong discriminatory power in distinguishing patients into high and low-risk categories. We also identified risk factors associated with poor adherence and examined behavioural and clinical factors common among patients in the high-risk deciles for personalized interventions in collaboration with the client.
Model Accuracy and Interventions
We evaluated the performance of our model using sensitivity, precision, F1-score, and Area under the Curve (AUC)
Metric | Development | Validation |
---|---|---|
TPR | 88% | 85% |
FPR | 21% | 30% |
TNR | 79% | 70% |
FNR | 11% | 14% |
Precission_P | 87% | 81% |
Precission_N | 81% | 76% |
AUC | 0.93 | 0.87 |
Precission_N | 84.7% | 79.4% |
Our Client Stories
Leading Public Hospital, Texas
AI in Clinical Decision Support for Early Sepsis Detection

Finarb helped in clinical decision support with an AI-Driven Real-Time Sepsis Monitoring Solution to accurately risk stratify patients who have a high likelihood of developing sepsis within a certain time window based on biomarker levels and vitals, sourcing data from Patient Monitoring Devices and Lab Information Systems.
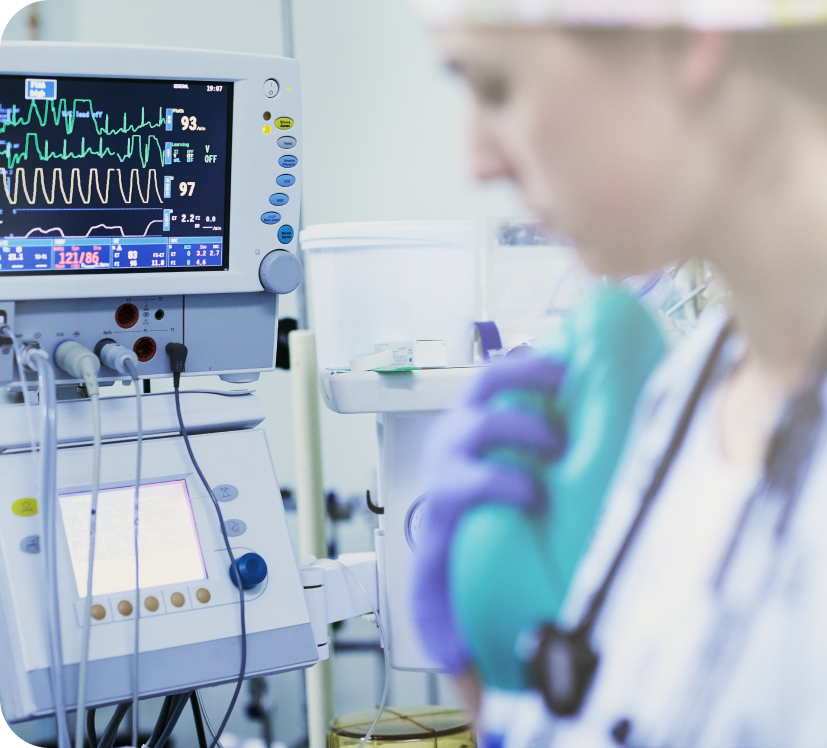
A leading pharmacy support services provider
Using Real World Data in AI driven Medication Adherence

Finarb collaborated with a leading pharmacy support service provider, to develop an AI enabled medication adherence monitoring model, integrating real world data & refill data, to accurately stratify high-risk patients, identify root causes of weak adherence and suggest precision targeted interventions.
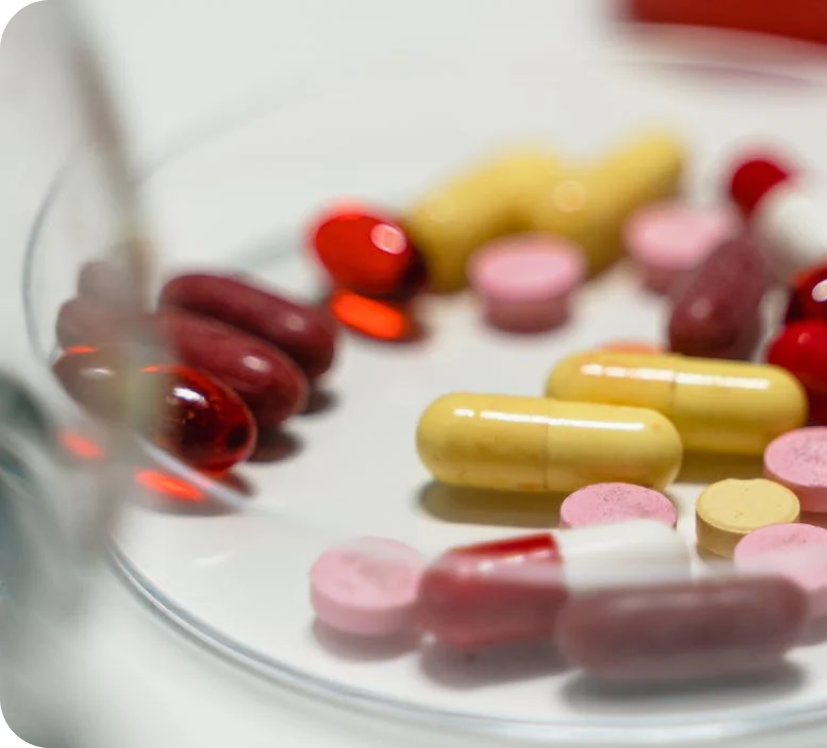
Radiology Clinic Chain, Texas
Tackling patient compliance with secure data warehousing

Finarb built a clinical data warehouse for a leading radiology clinic based in Texas, operating 100+ centers in 13 markets. With data spread across disparate sources, the clinic was struggling with patient compliance and low room utilization. We enabled secure integration of data from multiple structured and unstructured data sources and healthcare IT systems, followed by predictive modelling to identify patients with a high risk of non-compliance and failure points with targeted interventions to improve future compliance.
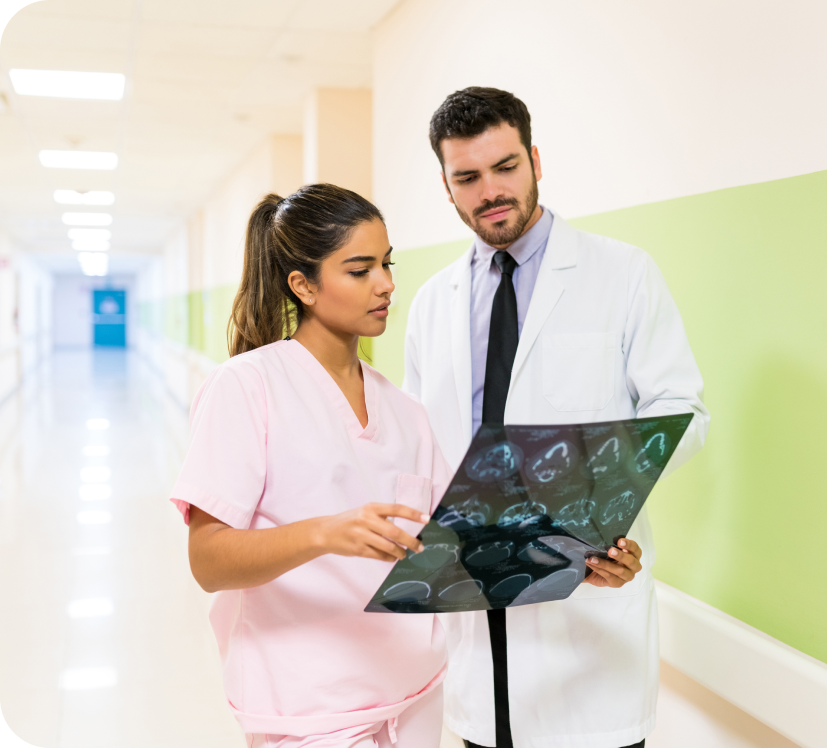