Dynamic Pricing AI: Solving Pricing Challenges and Supply Shortages
TABLE OF CONTENTS
How Dynamic Pricing AI might be the answer to pricing challenges and supply shortages?
In recent years, dynamic pricing has gained momentum in the pharmaceutical sector. With the increasing complexity of healthcare systems and escalating costs of research, development, and distribution, pharmaceutical companies are facing intense pressure to maintain profitability. Consequently, concerns about the economic sustainability of generic medicines have surfaced in the US market with the looming threat of supply disturbances and shortages. This is where AI-powered dynamic pricing emerges as a transformative force, promising to revolutionize how medications are priced, distributed, and accessed.
As prices of medications surge due to drug shortages, accessibility for many is compromised, sparking ethical concerns. Dynamic pricing, rooted in accurate demand forecasting, offers a strategic avenue to address supply chain challenges. By adjusting prices based on demand and supply fluctuations, this approach encourages suppliers to efficiently manage shortages, preventing sudden spikes in drug prices while maintaining affordability for consumers. Before implementing dynamic pricing, factors such as market demand, competition, regulatory compliance, and ethical considerations are meticulously evaluated. However, the adoption of dynamic pricing in pharmaceuticals demands a delicate balance, especially considering the ethical implications. Unlike consumer goods, drug pricing must navigate a complex landscape, to ensure ethical standards are upheld and access to essential medications is maintained, particularly for patients with limited financial means.
What are the different types of Dynamic Pricing?
Within the pharmaceutical industry, a resilient dynamic pricing solution encompasses various driver modules operating collaboratively to formulate price recommendations for the entire product portfolio. Developing a dynamic pricing strategy involves a multi-step process that engages various stakeholders in the value chain. Initially, producers or pharmaceutical companies assess various factors, including production costs, market trends, and competitive landscapes, to formulate a pricing model. Distributors then contribute valuable insights into market demand, inventory levels, and distribution costs. Subsequently, retailers collaborate with distributors to fine-tune pricing strategies, leveraging their understanding of local consumer behavior and preferences. As the product reaches consumers, their purchasing patterns and responses to pricing adjustments provide crucial feedback. Continuous monitoring of market dynamics, competitor pricing, and consumer feedback allows for real-time adjustments, often facilitated by algorithmic tools. Let's take a look at a few of the crucial dynamic pricing strategies and understand each of their functionality.
Demand-Based Pricing Model
Demand forecasting plays an important role in guiding businesses to make informative decisions about their medication pricing strategies. It is the process of analyzing historical data to build models that help businesses predict future scenarios related to product prices, product roadmaps, and consumer demand dynamics over a certain period. By understanding the market conditions, and historical sales, companies can predict customer demand, and optimize their pricing to maximize revenue and stay competitive instead of setting fixed prices. In the pharmaceutical market, dynamic pricing can be useful in scenarios where demand also follows a seasonal pattern. For instance, during the winter months, prices can be adjusted to meet increased demand for medications related to respiratory conditions. Similarly, during monsoon seasons, pricing strategies can be adapted to manage demand for medicines addressing waterborne or vector-borne illnesses. This responsive approach ensures a balance between supply and demand, preventing shortages and ensuring the availability of essential medications during health-related peaks.
Real-time monitoring allows quick adjustments, making dynamic pricing a flexible and effective strategy for addressing seasonal demand fluctuations in the pharmaceutical sector. By using advanced forecasting techniques like Classic Time Series and ARIMAX, alongside real-time data, relevant features could be extracted to build a robust and comprehensive demand forecasting model that would address the nuances of dynamic pricing. The resulting framework not only supports effective decision-making but also enhances demand planning, mitigates inventory risks, and amplifies sales volume, culminating in an overall boost to profitability for businesses.
Dynamic pricing based on Customer Pricing Sensitivity
Price sensitivity, or price elasticity of demand, refers to how the demand for a product changes in response to price changes. In the generics industry, the implementation of dynamic pricing based on customer pricing sensitivity is intricately tied to the concept of price elasticity. Understanding the elasticity of demand for pharmaceutical products is crucial for tailoring effective pricing strategies. The customers primarily refer to institutional entities such as hospitals, healthcare providers, and pharmacies. The pharmaceutical market is often considered to have elements of both elastic and inelastic demand. Medications addressing critical conditions often exhibit inelastic demand, where consumers are less responsive to price changes due to the essential nature of the treatment. For these products, dynamic pricing involves careful considerations to minimize revenue losses, recognizing that consumers are less likely to alter their purchasing behavior even in the face of price increases. Conversely, pharmaceutical goods for less severe conditions, with available alternatives, may experience elastic demand, rendering consumers more price-sensitive. In such cases, dynamic pricing strategies can be leveraged to adjust prices based on market conditions, competitor actions, and customer preferences. The following mathematical formula is used to calculate the price elasticity of demand.
For instance, if the price of a product increases from $10 to $12 (a 20% increase), and the quantity demanded decreases from 100 to 90 units (a 10% decrease), the PED would be calculated as: PED= (−10%)/20%= −0.5
A negative PED is typical, signifying an inverse relationship between quantity demanded and price. Here, a PED of -0.5 indicates inelastic demand, as the magnitude is less than 1, implying that the percentage change in quantity demanded is less than the percentage change in price.
Competitor-Based Dynamic Pricing
Competitor-based pricing is a strategy where businesses set their prices by analyzing market rates and studying the pricing strategies of competitors. This approach involves monitoring and collecting data on competitor prices through market research, industry reports, web scraping, social media monitoring, collaboration with distributors, and internal sales analytics while ensuring ethical and legal compliance. The pricing adjustments can be influenced by factors such as competitor pricing changes, market share considerations, and the introduction of new products or treatments. For instance, If a competitor lowers the price of a specific generic medication, a company may opt to match or undercut that price to stay competitive whereas, if a competitor increases the price of a generic drug, other companies may assess their own product portfolio and pricing strategies accordingly to maintain their competitive position.
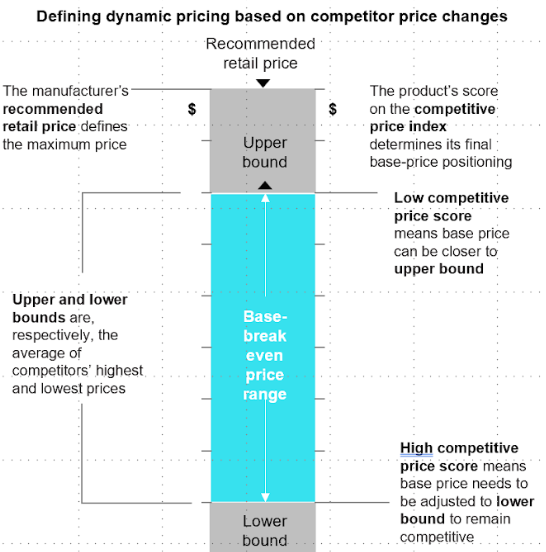
How to implement dynamic pricing models?
To implement an effective dynamic pricing model, companies utilize machine learning (ML) algorithms and forecasting techniques. ML algorithms, such as regression analysis and neural networks, analyze vast pricing datasets, to analyze vast sets of pricing data, identifying patterns and relationships between factors like product features, brand reputation, and competitor pricing. Time-series forecasting, like ARIMA, helps predict future demand, allowing proactive price adjustments. The integration of ML in competitor-based pricing ensures accurate predictions for dynamic adjustments, balancing competitiveness and profitability.
In summary, the synergy of ML algorithms and forecasting techniques empowers companies to dynamically set prices, respond to market changes, maximize revenue, and stay competitive.
The Bottom Line
Dynamic pricing proves to be invaluable across the pharmaceutical industry. However, manually configuring prices is a laborious task, susceptible to errors, and demands a significant investment of staff hours. In the realm of Artificial intelligence, AI-driven Dynamic Pricing emerges as a powerful mastermind in optimizing pricing strategies, enhancing competitiveness, and responding dynamically to market dynamics.
By leveraging real-time data analytics and machine learning algorithms, pharmaceutical companies can navigate the complex landscape of price elasticity, competitor actions, and customer behavior. The judicious application of AI-driven Dynamic Pricing not only facilitates revenue optimization but also ensures that critical medications remain accessible, reinforcing a delicate balance between commercial viability and societal well-being. As technology continues to advance, the pharmaceutical sector stands to benefit from the adaptive and intelligent capabilities of AI in shaping a more responsive and efficient pricing ecosystem.