Utilizing Real-World Data to Address Medication Persistence
TABLE OF CONTENTS
Medication Persistence: A Vital Healthcare Focus
In healthcare, medication persistence represents the duration of time from initiation to discontinuation of a prescribed medication regimen. It reflects the extent to which patients adhere to their treatment plans over time. Low medication persistence is a serious concern that not only affects the clinicians but also the healthcare systems and other stakeholders. medication persistence analysis primarily assesses the duration over which a patient continues to take their prescribed medication without unnecessary interruptions. The consequence of medication non-persistence leads to adverse outcomes like waste of medication, worsening of disease, reduced functional abilities, lower quality of life, increased use of medical resources, or even death. The potential disadvantages of medical non-persistence outcomes in health care systems make it an important public health concern.
Deciphering the Complexities of Medication Persistence Using Real World Data
In the intricate domain of medication persistence, understanding patient behavior toward their regimen is paramount. The three primary types of models commonly used in analyzing medication persistence are:
- Adherence: This metric, often quantified using the Medication Possession Ratio (MPR) or Proportion of Days Covered (PDC), gauges how consistently patients stick to their medication plan.
- Switching: Insights into why patients change medications—whether due to inefficacy, side effects, or other factors—are crucial in understanding the evolving therapeutic landscape.
- Abandonment: Identifying the reasons patients discontinue therapies can reveal both broader systemic challenges and individual barriers.
Rethinking Traditional Medication Persistence Tracking in the Age of Digitalization
Medical persistence tracking has heavily relied on historical data, and this data is utilized and validated in various conditions and settings following different approaches. The methods of assessment are mainly classified into traditional methods vs new age solutions. Within the traditional methods fall the measurement of drug or metabolite concentrations such as blood tests, urine drug testing, or through the evaluation of the presence of a biological marker related to the drug. While these methods offer precision and the margins of error are minimal, their feasibility for widespread and frequent use is constrained by factors such as cost, invasiveness, and logistical challenges. More critically, it doesn't possess the capability to project future persistence based on past behaviors.
The pressing need for alternative approaches that can provide reliable medication persistence monitoring across a broad population base, enabling more comprehensive assessment and intervention strategies led to the adoption of AI-driven approaches that use a combination of pharmacy data, claims data but also integrates real-world data collected through patient surveys, self assessments or through wearables and patient monitoring apps, to accurately measure and monitor not only current persistence levels but also predict future risk based on past behavior with mitigation measures for control.
Unlocking the Potential of Real-World Data in Medication Persistence Analysis
Real World Data (RWD): A Brief Review
Real world data is the raw data relating to a patient's health collected from a variety of sources including EHRs, insurance claims, bills, product and disease histories, etc. A critical component of real world data collection is direct patient assessments, which offer detailed insights into individual patient experiences and behaviors. Moreover, the integration of data from health monitoring or wearable devices has revolutionized real world data, offering insights into individuals' health metrics. While traditionally real world data has been used in clinical research for cohort studies, in assessing clinical trial effectiveness and post marketing surveillance to monitor the safety and effectiveness of drugs or medical devices, today, the acquisition and analysis of real world data has taken a more central role in understanding and monitoring medication persistence.
It’s easy to see why - an individual's adherence to a medication regime can be significantly influenced by real-world factors such as socioeconomic status, education level, and access to healthcare facilities. Real world data helps in capturing such nuanced information, providing a comprehensive picture of medication persistence across different demographic groups and settings.
Navigating Challenges Related with Real World Data Analysis
Although real world data offers transformative insights into healthcare, its messy and heterogeneous nature can impair its utility. Various challenges ranging from data gathering to data quality control to decision making still exist in all stages of a real world data life cycle which diminishes all the enthusiasm around its transformative potentials. Issues related to data sparsity, data quality control, sampling biases etc are the common ones. Here we are listing a few challenges related to real world data analysis, where plenty of opportunities for improvement exist and greater efforts are required to harness its power.
- Sampling Issues & Data discrepancies faced due to Survey Dropouts: When collecting data, it is important to determine the right sample size and the correct respondent group. Every researcher aims for a sample that addresses the larger population but often ends up with non-respondents or a smaller response group. For example, you are aiming for a batch size of 200 to fill your survey questions, but only 100-120 end up filling the survey. It generally occurs when a sample is not representative of the population or is not interested enough in the survey. Besides this, individuals might even leave the survey midway without completing it, resulting in survey dropouts. A high Dropout rate (DoR) compromises the representativeness of your survey audience. Survey dropouts aren’t just numbers that indicate incomplete responses; instead, they result in incomplete data collection and data gaps which often lead to misinterpretations thereby delivering erroneous conclusions and insights. Another drawback of Survey dropout is that it leads to waste of time, resources, and campaign costs. Companies spend a lot of time, resources, and even incentives trying to strategize a good survey that would fetch them accurate answers, but dropouts prevent the ultimate aim. These issues could be mitigated through optimum incentivization to ensure survey completion, improved survey design and communication, follow-up strategies, and ethical considerations.
AI driven approach for Enabling Efficient Survey Completion through Optimum Incentivization: Finarb and Apollo Intelligence Case Study
One solution formulated by Finarb aimed at enabling sufficient survey completion and reducing survey costs. In this case the survey takers were doctors. The aim was to understand the likelihood of doctors filling the survey, and providing an optimal incentivization schedule for surveys which would help achieve a predetermined number of filled surveys at minimal cost. A classification model was built on the data gathered from previous surveys. The data set had information on the amount of incentives offered, whether a survey was completed or not, information on reminders sent, demographic details of the doctors, their medical background, etc. Feature engineering was performed on the dataset to extract other relevant features such as no. of emails sent to a doctor, time taken to complete the survey, and historical interaction of the doctors with the survey platform. The classification model estimated probability of a doctor completing the survey, and also calculated optimal value for initial incentive, no. of reminders sent, delay between reminder touchpoints & incentive amount step ups needed. Find more details about the case study here.
- Lack of Standardization & Reliability: When collecting real-world data, lack of standardized formats and coding systems across different sources make it difficult to integrate and analyze real-world data effectively leading to quality issues like duplicate, missing, and outlier data. With real world data originating from patient assessment surveys, wearables, EHR, pharmacy refill data, claims data and others, it is difficult to combine data without losing information because each database has its own original objectives, purpose, structure, and terminology. Standardization requires an industry-wide dialogue to develop common data collection and analysis which would facilitate better research and improve the quality of data. A logical solution to address this problem would be to store data in a standardized format, such as a common data model (CDM) developed by the Observational Health Data Sciences and Informatics (OHDSI) community - this allows transformation of data contained in different databases to a common format. This standardization helps in mixing diverse data sources, ensuring consistency and reliability in real-world data studies.
Data Cleaning: The Non-Negotiable Pillar of Real World Data
While using the CDM developed by OHSDI improves an organization's analytic capacity in multiple ways, it is still not widely used by healthcare firms possibly due to lack of awareness of such frameworks, which means the industry would need to be thorough with their data cleaning measures to deal with any data quality issues including duplicate records, inconsistent or incorrect data, data gaps, outliers, all of which can severely impede results of data analyses and provide wrong insights, and can prove to be grave for an industry like healthcare. This is when data cleaning comes into play.
Data Cleaning is the process of identifying, diagnosing, and fixing inconsistent data. This involves correcting errors, removing duplicates, and handling outliers. Since the amount of data across healthcare is greater than in most other industries, cleansing ensures that healthcare information continues to be accurate. The unprocessed raw data from the source is first collected, following which the data cleaning rules are applied using appropriate data-cleaning frameworks and cleaning algorithms. Data Wrangler, OpenRefine, Python, and R are some commonly used tools in the data-cleaning industry. Furthermore, data imputation techniques also play a pivotal role in addressing missing or incomplete data within datasets. These methods involve predicting and filling in missing values, enhancing the overall completeness and reliability of the dataset. Various approaches, such as mean or median imputation, regression imputation, or advanced machine learning-based methods, are employed based on the nature and context of the missing data. By strategically imputing missing values, organizations can ensure more robust analyses, improve model performance, and derive more accurate insights from their datasets.
- Addressing the Challenge of Integrating Unstructured Patient Assessment Survey Data into Structured Datasets - The Role of NLP Tools: Another crucial aspect to consider is the prevalence of unstructured real world data, particularly evident in the format of many patient surveys and Healthcare professional surveys. The key solution lies in harnessing Natural Language Processing (NLP) techniques to extract meaningful insights from this unstructured data, subsequently transforming it into a structured format. This approach not only ensures the efficient handling of diverse survey formats but also unlocks valuable information hidden within the unstructured data, providing a more comprehensive and accessible foundation for analysis and decision-making.
Spotlight: Collaboration between Finarb and a leading pharmacy support service provider - Using Real World and Pharmacy Data to improve Medication Persistence (in this case we measured Medication Adherence)
The Real-World Challenge That Prompted the Use of AI in Medication Adherence
The Client, being one of the largest providers of pharmacy support services in the US works with a lot of hospitals and pharmacies providing automated patient care solutions that improve patient experiences and health outcomes. The Client wanted to develop a solution to tackle the risk of medication non-adherence by patients as it directly impacts the results of treatment plans and results in sub-optimal health. The traditional methods for improving adherence produced inconsistent and inaccurate results which prompted the use of AI-enabled medication adherence monitoring to accurately stratify high-risk patients and suggest precision targeted interventions to improve adherence and the overall healthcare system efficiency. Read the detailed case study here.
Fusion of Real World Data with Clinical Data Inputs
The Client wanted to create a solution to address the pervasive issue of medication non-adherence. To effectively develop this solution, it was necessary to identify the underlying causes of weak adherence and recommend precise, targeted interventions to enhance adherence levels. The initial phase involved gathering real-world patient assessment data through the implementation of primary data collection surveys and questionnaires. A standardized questionnaire was uniformly distributed by the client to all participating pharmacies, which served as the instrument for collecting patient feedback. Recognizing the need for consistency, the questions were categorized into distinct local groups such as "Adherence," "Adverse Event," "Barriers," "Benefits," "Patient Profile," and more.
Within the "Adherence" category, questions delved into variations ranging from missed doses to the reasons behind missing doses or the quantity of remaining doses. The "Adverse Event" group focused on inquiries related to any side effects experienced, the severity of these side effects, and the overall outcomes. Additional categories like "Benefits" explored questions related to both short-term and long-term benefits observed by the patients, as well as inquiries regarding other therapeutic benefits or outcomes. This categorization process was a collaborative effort with the client LLC, ensuring that the groupings aligned with the business perspective. The logical grouping of questions is mentioned below.
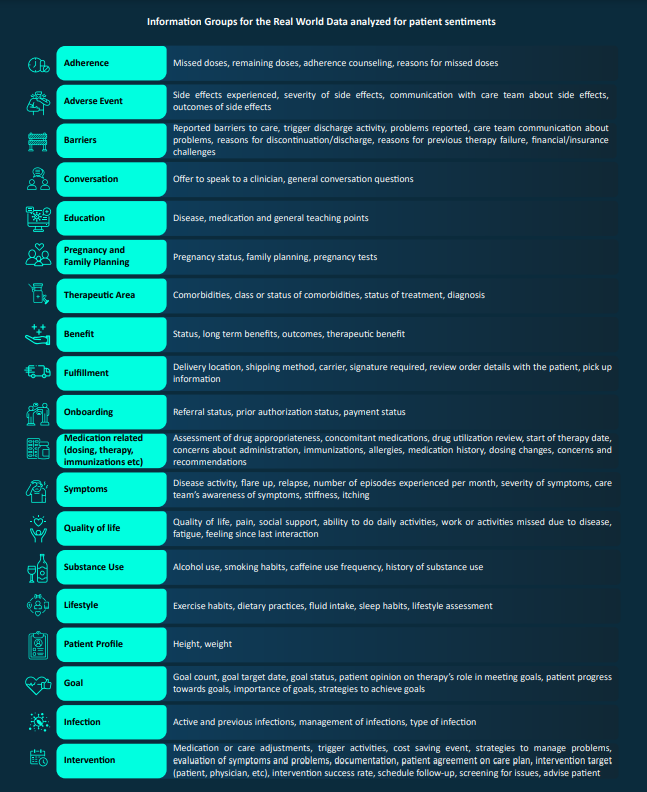
The real-world patient assessment data acquired through these surveys and questionnaires were then integrated with medication refill data sourced from pharmacy dispensing databases. An extensive analysis was conducted on approximately 1.8 million data points, representing patients who had been on medication for a minimum of 180 days, with an average of six refills and two follow-up assessments. It is noteworthy that all Protected Health Information data underwent encryption following best practices before the model training commenced.
Exploratory Data Analysis and AI modeling to arrive at future predictions of non-adherence
The primary aim was to construct an Adherence Risk Model for stratifying rheumatoid arthritis patients based on non-adherence risk scores. The objectives included identifying key determinants of adherence, pinpointing high-risk patients, and addressing specific areas of concern. Medication adherence was measured using (PDC), the Proportion of Days Covered. A PDC score above 0.9 denoted adherence, while a score below 0.9 indicated non-adherence.
Before developing the Adherence Risk Model, an extensive Exploratory Data Analysis (EDA) phase was diligently carried out. This involved comprehending data structures, trends, and patterns to select features and model building. During EDA, outliers were addressed to enhance model accuracy, and data imputation methods were applied to rectify missing values. Integration of public datasets such as the US Census, NPI registry, and Zipcode latitude-longitude data was performed to enrich the dataset. Ensuring that AI models held no prior assumptions about patient adherence based solely on outcome or refill data was a crucial step, and measures were taken to prevent algorithmic bias, considering factors like Social Determinants of Health (SDOH) and gender disparities in medication refill behavior.
Following EDA, an exhaustive list of features influencing medication adherence was identified and analyzed. This encompassed patient demographics, prior adherence performance, SDOH data, and medication features (e.g., drug name, strength, route of administration). New potential predictive variables were also introduced. To enhance accuracy and interpretability, patient sentiment analysis and Natural Language Processing (NLP) techniques like BERT were employed to analyze unstructured patient feedback data from follow-up assessments.
The feature importance method was employed, revealing the top 10 features. The number of previous follow-up assessments emerged as the most influential predictor of medication non-adherence, followed by the average PDC until the last refill and symptoms question group. These features were utilized in predicting non-adherence using the 'Random Forest' machine learning classifier, known for its ability to discern both linear and non-linear relationships between explanatory and dependent variables. The model underwent training and hyper-parameter tuning to strike a balance between bias and variance, learning relationships between features and medication adherence status, providing a probability score for a patient's future PDC.
Risk stratification was performed through decile analysis to categorize patients into high and low-risk groups based on predicted adherence levels. Deciles with capture rates of 60% or higher were identified as high risk, primarily composed of non-adherent patients. Remarkably, 80% of patients could be confidently segmented into either high or low-risk groups, showcasing the effectiveness of the risk stratification conducted through the model.
The Client is currently evaluating ongoing improvements over time, focusing on identified risk drivers for high-risk patient cohorts as identified by Finarb. The interventions implemented target the enhancement of medication adherence through promoting transparent communication and reinforcing the therapeutic importance of prescribed medications. Through a thorough analysis of the outcomes of these interventions on the identified cohorts, the client and Finarb aimed to determine the effectiveness of specific actions on patient outcomes. This implementation is anticipated to bring about a significant enhancement in patient outcomes and healthcare efficiency, aligning with the client's vision to revolutionize community healthcare. A notable 35-40% reduction in the risk of non-adherence is anticipated, starting from an initial risk of 42%, within the first 6-12 months of deploying the model.