Revolutionizing Healthcare with AI in Sepsis Detection: A Case Study
AI-driven sepsis detection reduces mortality by 37.54%. Real-time monitoring and predictive models enhance patient outcomes and efficiency
Overview
- Client: A leading Public Hospital in Dallas, Texas
Business Goal :
- Early Detection of Sepsis
- Improving Patient Outcome
- Enabling real-time clinical decision support systems
- Increase operational efficiency.
Business Outcome:
- 37.54 % reduction of in-hospital mortality
- 34.13% reduction in hospital Length of Stay
- Real-time monitoring and alerts
-
Improved Accuracy
and Enhanced Workflow
AI in Sepsis Detection: Transforming Healthcare for Early Disease Diagnosis and Prevention
In the ever-evolving healthcare landscape, timely detection of life-threatening conditions is paramount for ensuring positive patient outcomes. The role of Artificial Intelligence (AI) in healthcare is becoming increasingly vital, particularly in the context of early disease detection and clinical decision support. This case study showcases the transformative power of AI, focusing on its application in early sepsis detection at a leading Public Hospital in Dallas, Texas. Let's delve into how AI is redefining disease diagnosis and prevention
Insights related to Sepsis in US
- According to the CDC, roughly 1.7 million adults in the U.S. develop sepsis each year, with a fatality rate as high as 20% in case of severe sepsis1
-
1 out of 3 hospital deaths in
US are a result of Sepsis2
- Sepsis, or the infection causing sepsis, starts before a patient goes to the hospital in nearly 87% of cases3 .
- In the US, Sepsis patients have the highest cost of hospitalizations, consuming more than $20.3 billion each year5.
- The average cost of a stay is estimated at $18,400 per admission5. Considering the mortality rates and economic impact associated with sepsis, timely detection of sepsis is crucial.
-
The risk of death for Sepsis
patients varies based on the stage
of Sepsis they are in. The table
below outlines the approximate
fatality rate for each stage4
The Solution: AI-Driven Predictive Model for early detection of Sepsis
To address this challenge, the client partnered with Finarb, a consult-to-operate advanced AI and data solutions provider, to develop a comprehensive solution. In close collaboration and consultation with the client's healthcare professionals (Lead doctors and nurses) and the IT team, a deep understanding of the clinical context, available data, type of data, clinically significant attributes, and crucial patient symptoms was gathered.
Data Collection and Encryption:
Automated ETL and Data Preprocessing:
Feature Selection and Feature Engineering to identify clinically relevant variables suggesting sepsis onset:
After processing the raw data, collaborative sessions were held with hospital doctors and healthcare experts to identify key sepsis-related variables. Additional features were engineered to enhance predictive accuracy. Advanced algorithms were then employed to determine feature importance for sepsis risk prediction.
- Variable selection - Identifying clinically relevant variables, including patient demographics, vital signs, laboratory results, and biomarkers (e.g., IL-6, TNF, IL-8, MCP-1, CRP, and PTX-3).
- Feature Engineering - Creating derived features, such as a 24-hour aggregate vital score (average, maximum, and minimum), to capture data patterns.
- Feature Importance - Determining feature importance using advanced algorithms to gauge their impact on sepsis risk prediction.
AI Modeling and Risk Score Prediction
The Sepsis Early Detection Algorithm (SEDA), developed by Finarb, predicts sepsis risk dynamically based on collected data. It can be customized for different timeframes (e.g., 72, 48, 24, 12, and 6 hours before sepsis onset) to match clinical needs. For example, if sepsis is predicted 48 hours ahead, it assigns a probability score for that timeframe. As the prediction window shortens, updated risk scores reflect changing sepsis risks. Patient risk scores are also updated in real time based on their current condition.
The threshold risk score for sepsis was a collaborative effort between the doctors, healthcare experts, and data scientists at client and Finarb, to establish a consensus regarding the threshold for identification of high-risk patients and subsequently in predicting early detection of sepsis. The ROC curve was plotted, which showed the true positive rate against the false positive rate. Based on discussions with the doctors regarding the trade-off between true positives and false positives, we arrived at the following threshold risk score, which classified the patients into high, medium, and low risk groups.
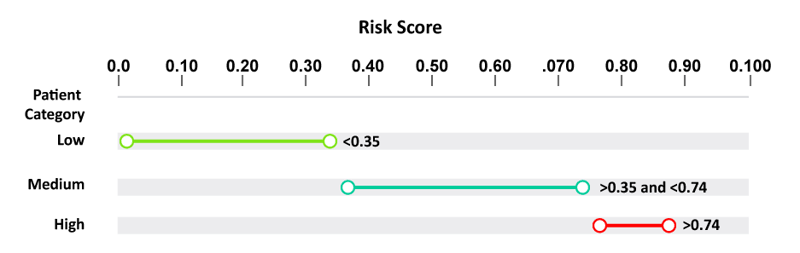
Building the web-based application for real-time Sepsis Detection :
A user-friendly web application was created for real-time monitoring of patient data, vitals, and risk scores. The application was built in compliance with SMART on FHIR guidelines and is customizable for any use case in preventive healthcare.
Key features of the application include:
- Comprehensive Dashboard: Integrates data from various sources, displaying real-time patient information, vitals, and risk scores. It allows healthcare professionals to monitor multiple patients, analyse historical data for trends, and track patient progress.
- AI-driven Predictive Modelling: Utilizes predictive models to identify early signs of sepsis by analysing data and providing real-time risk scores, offering actionable insights.
- Alert Generation: Detects abnormal values or trends in vital signs and laboratory results, triggering notifications for timely intervention by healthcare providers or rapid response teams for priority patients.
Results and Benefits:
The collaboration between Finarb and the client in developing the predictive model and Sepsis Monitoring Application has resulted in several notable benefits:
- Early Sepsis Detection: By continuously analyzing real-time patient data and assigning probability scores to patients, doctors and the rapid response team could proactively intervene and initiate timely treatments, significantly reducing the risk of complications and mortality. The average reduction in mortality was 37.54%, and the average length of Stay of Patients in the Hospital’s ICU unit decreased by 34.78 % (the data was taken for 179 patients)
- Real-time Monitoring and Alerts: A real-time centralized application for monitoring patient vitals and parameters. Any changes were seamlessly displayed and flagged, if needed, with an alert system for healthcare professionals.
- Improved Accuracy: The model had a high AUC-ROC score of 0.90. The accuracy obtained was for a time window of 24 hours. As the time window narrowed, the AUC-ROC score reached 0.94.
- Enhanced Efficiency and Workflow: The application optimizes healthcare efficiency by enabling monitoring of multiple patients simultaneously and improving resource allocation.
AI in sepsis detection is not just a buzzword; it's a transformative force reshaping the healthcare landscape. This case study demonstrates how AI can revolutionize early disease detection, diagnosis, and preventive healthcare. The success story of this Public Hospital in Texas and Finarb underscores the potential of AI to save lives and improve patient outcomes. As the healthcare industry continues to embrace AI, the future holds promising possibilities for early disease detection and prevention.